在Journal of the American Statistical Association期刊上,杨玥含教授与清华大学的刘汉中副教授以及在读博士任吉杨合作发表了围绕多因子随机区组实验的因果推断论文。该论文针对此类实验提出了新的随机化中心极限定理及相应的有效协变量调整方法。随机区组实验在工业工程、临床试验和社会科学等多个领域被广泛应用,该论文为随机区组2K因子实验的常规(未调整)因子效应估计量建立了一个新的有限总体联合中心极限定理,该定理在一个基于随机化的推断框架下获得,利用了线性秩统计量的Wald-Wolfowitz-Hoeffding定理向量形式的扩展,对模型错配、区组数、区组大小以及跨区组的倾向性评分均具有稳健性。为了提高估计和推断的效率,该论文提出了四种协变量调整方法,并证明了这些协变量调整因子效应估计量是一致的、联合渐近正态的,并且通常比未调整的估计量更有效。此外,该论文提出了Neyman类型的保守估计量来估计渐近协方差,以便于进行有效的推断。
论文题目:Randomization-based joint central limit theorem and efficient covariate adjustment in randomized block 2^K factorial experiments
论文摘要:Randomized block factorial experiments are widely used in industrial engineering, clinical trials, and social science. Researchers often use a linear model and analysis of covariance to analyze experimental results; however, limited studies have addressed the validity and robustness of the resulting inferences because assumptions for a linear model might not be justified by randomization in randomized block factorial experiments. In this paper, we establish a new finite population joint central limit theorem for usual (unadjusted) factorial effect estimators in randomized block $2^K$ factorial experiments. Our theorem is obtained under a randomization-based inference framework, making use of an extension of the vector form of the Wald--Wolfowitz--Hoeffding theorem for a linear rank statistic. It is robust to model misspecification, numbers of blocks, block sizes, and propensity scores across blocks. To improve the estimation and inference efficiency, we propose four covariate adjustment methods. We show that under mild conditions, the resulting covariate-adjusted factorial effect estimators are consistent, jointly asymptotically normal, and generally more efficient than the unadjusted estimator. In addition, we propose Neyman-type conservative estimators for the asymptotic covariances to facilitate valid inferences. Simulation studies and a clinical trial data analysis demonstrate the benefits of the covariate adjustment methods.
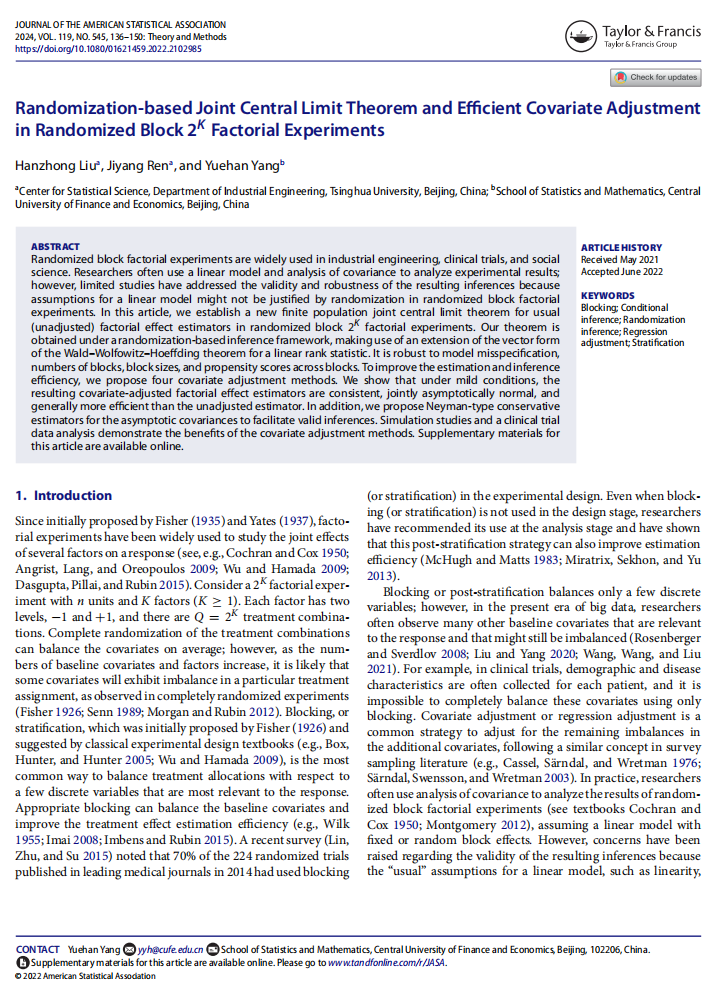
作者介绍:
杨玥含,十大澳门信誉老牌网赌教授,龙马青年学者,主要从事复杂数据建模、因果推断、迁移学习等研究,作为独立作者、第一作者或通讯作者在统计学四大期刊Journal of the American Statistical Association、Biometrika、人工智能顶级期刊Pattern Recognition、Expert Systems With Applications、Knowledge-Based Systems等期刊发表论文近40篇。
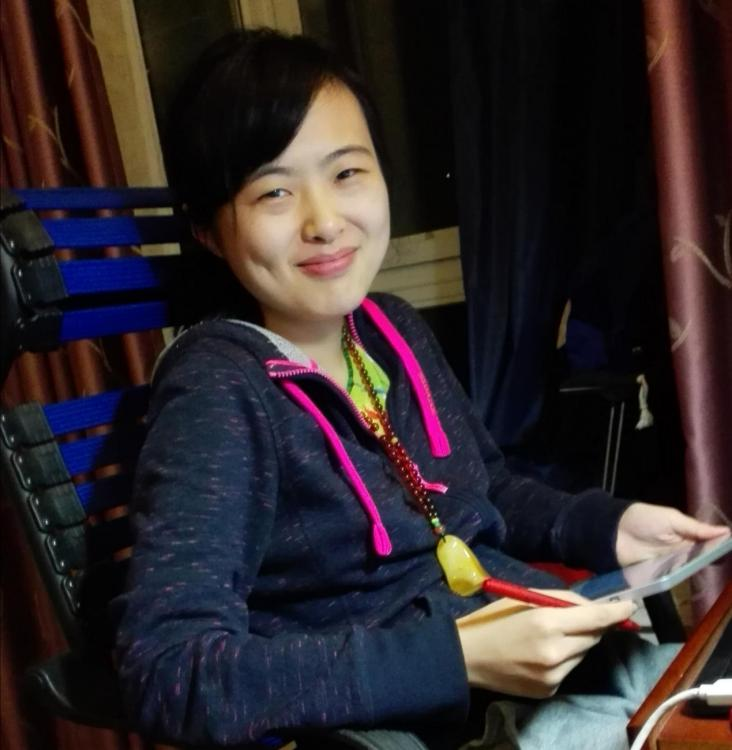